Analytics implementation and measurement: Getting it right
Written by Ian Cockayne
This is the third in our series of eight data engineering and insights articles in which we delve into analytics implementation and measurement. We will explore the common mistakes that cause tracking errors and data quality issues and how to mitigate for them to collect data reliably so that we can confidently make decisions based upon robust data.
From observed data to modelled data
Before digging into the detail, it is also important to remember that the landscape of data analytics has evolved significantly over the last decade, including multi-device, multi-session user journeys and the increasing prevalence of privacy-preserving technologies which limit the visibility that marketers have of their visitors.
Traditionally, web analytics relied heavily on direct observation of user behaviour such as sessions, page views and clicks. However, as technology has advanced and the number of data gaps have increased, a more nuanced approach has evolved. This leverages data-modelling techniques powered by advancements in machine learning and artificial intelligence to extrapolate and predict behaviours based upon patterns and correlations from data that has been collected in order to bridge data gaps.
Big data does not work without the little data
There is often a lot of hype surrounding subjects such as big data, machine learning and currently artificial intelligence; the quality of the modelled data that these tools create is only as reliable as the data they use to learn from in the first place. With that in mind, whilst a proportion the data that we use for making decisions will have been generated in some way, we need to ensure that the data we do collect is as robust as possible which means still doing the analytics implementation essentials right.
Common mistakes in analytics implementations
1. Lack of prioritisation
In our first data article, we talked about aligning business goals with digital analytics by defining Key Performance Indicators (KPIs). Implementing analytics without a focus on your important interactions is starting off on the wrong path, and in doing so, is likely to lead to more of the errors that we will see below. So, it is critical that you start out with your KPIs and regularly audit your setup in the future to ensure that the KPIs that are tracked are in step with your current business needs, whilst weeding out unnecessary data points that do not add value.
2. Lack of documentation
It still surprises me how often I encounter an analytics implementation with no supporting documentation whatsoever. A tracking specification is a blueprint that outlines what data needs to be tracked, how it should be tracked and the intended use of this data. Without a clear specification the tracking setup is going to be prone to inconsistencies and gaps along with there being no evidence of what good looks like to validate against.
3. Reliance on out-of-the-box installation
Another common issue is the over-reliance on out-of-the-box implementation or migration solutions provided by web analytics tools or other software vendors. Whilst these methods may simplify implementation, they will often rely on default settings which often results in suboptimal tracking. Out-of-the-box installations usually cover basic metrics but may not capture specific interactions crucial to the business, for example ecommerce tracking, user journey progress or custom event tracking which require custom configuration. Again, aligning your implementation to your business objectives through your tracking specification helps you define the customisations that have be made to get the insight you need.
4. Too much / ad-hoc tracking
On the other end of the spectrum, many agencies and organisations alike fall into the trap of tracking too many data points. In the world of digital, the emphasis is generally on creating new things, so a new shiny addition to a website generally means new shiny data points, regardless of whether they add true value, call it a shiny new object tax. Similarly, many organisations collect data with a just-in-case mentality, when in a privacy-focused world, we should be minimising our data collection to data that we are going to use and in turn minimising our exposure to privacy compliance issues. Too much tracking not only complicates analysis, it can also slow down your website performance due to excessive tracking scripts. Prioritising KPIs and focusing on the most impactful data points will keep your tracking set up lean, eliminate redundant data and save you time and money.
5. Unreliable Tracking Mechanics and Configurations
The business of tracking has greatly increased in complexity over the past decade since tools like Google Tag Manager came about. In a world without Cookie Banners and Content Security Policies, tag managers were a quick way to load tracking scripts on websites without the delay of developer intervention. This meant that tag managers could quickly become repositories of hordes of tracking nastiness such as incorrect tagging, missing or out-of-date tags, poor data layer logic and custom Javascript, copied from third party sites, often undocumented and unaudited. As tracking evolved, more complex mechanics have become a necessity such as Consent Banner logic and more recently, Server-Side tracking and more opaque data collection practices such as Consent Mode or Enhanced Conversions have added additional layers of complexity. The rigor that is required to maintain a privacy-compliant, performant analytics implementation necessitates thorough documentation, robust testing procedures and intentionally regular audits to ensure that the right tracking is optimised and that low-value or just plain sketchy data collection is (dare I say it) removed.
6. Lack of scrutiny
When auditing tracking implementations, aside from overcoming the disappointment of having no documentation to refer to, the second most common realisation I have is “Did anybody test this before they put it live?”. This is often the case when working with undocumented implementations that may have had several custodians which are often replete with ad hoc tagging in different implementation styles. Don’t get me wrong, ad hoc tagging can sometimes have its place, for example to strictly support some data collection for the life of an A/B test whereupon it is removed. However, on enterprise implementations ad hoc tagging often leads to data quality errors which would have been avoided if the implementation had been thoroughly tested. However, as this type of tracking is undocumented the data quality errors only become apparent after some serious investigation. This is why good quality analytics implementations are fortified through having robust testing in place to ensure that tracking is validated against a specification alongside stress-testing the rigor of the logic to ensure its robustness, alongside a regular audit process to re-validate tracking on a regular basis to ensure that the implementation continues to collect data reliably.
7. Lack of training
There are two aspects to this point. The first is the obvious one in that whoever is responsible for managing your analytics implementation should be trained in that field. Gone are the days that you will get away with being able to follow a few simple bullet points or screengrabs, implementing robust data analytics requires foundational knowledge as well as keeping up to date with innovations in the field, even if just to evaluate them against tried and tested methods to understand where they can be exploited in a way that is relevant to the solution that you are working upon. The second is the less obvious one of training on the documentation and setup of your existing implementation. Enterprise implementations are often a sprawl of interrelated tag managers and data platforms that can take weeks or months to become fully conversant with their configuration and idiosyncrasies. In both cases access to sufficiently detailed documentation, training and time to practice are key in building competence in your implementation team.
Conclusion
Whilst analytics implementation has evolved considerably and businesses are increasingly leveraging modelled data alongside their directly collected first-party data in their decision-making, technical failures in your tracking and measurement tools can severely impact your data quality and undermine your efforts.
By addressing common issues such as a lack of prioritisation, insufficient documentation, over-reliance on out-of-the-box solutions or unwieldy tracking mechanics, businesses can significantly improve the accuracy and reliability of their data collection. Supporting your analytics implementation with best practices and regular audits ensures a robust and effective analytics setup, providing valuable insights that drive informed data-driven decision making.
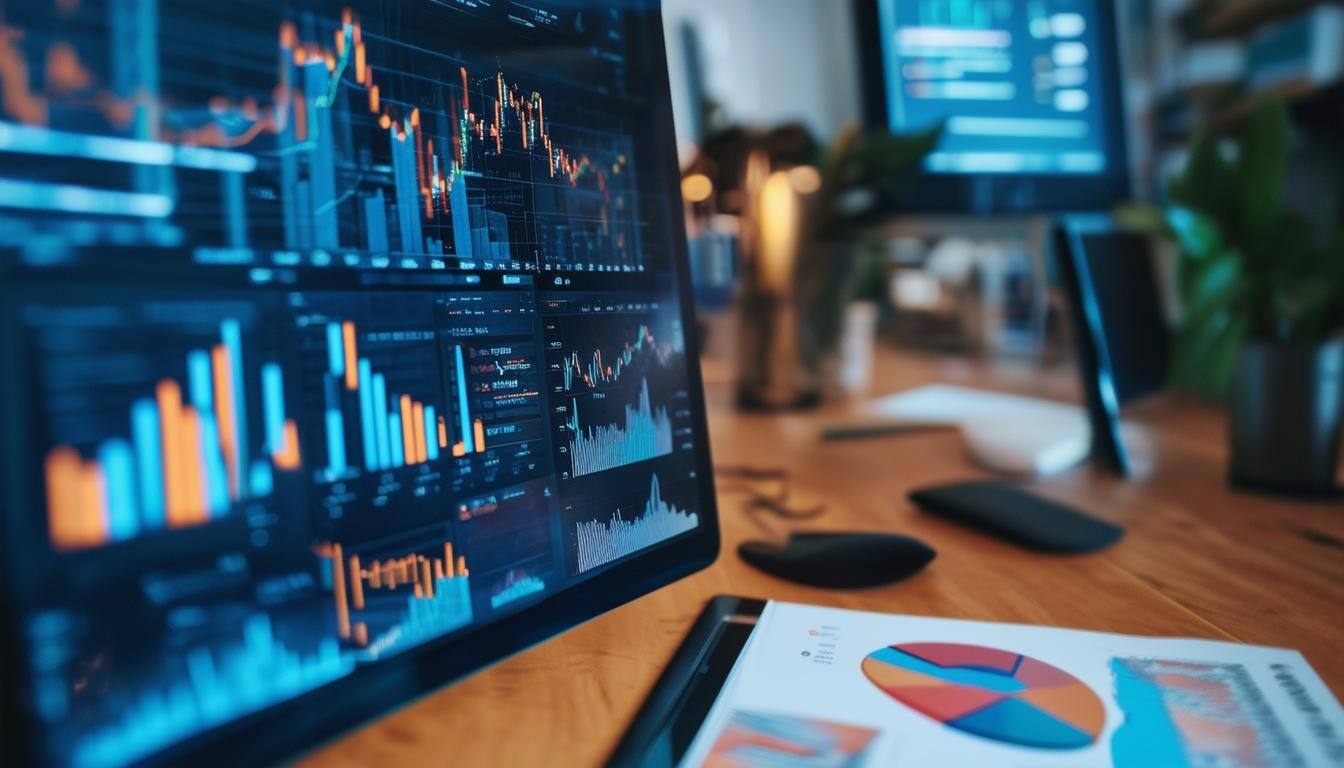
If any of this sounds familiar, or you think that your analytics implementation could do with a tune-up, contact us at Mando Group today to find out how we can help you.
What else is going on
-
March 2025
The Case for Migrating to Optimizely in 2025
Explore the real-world business case for switching to Optimizely. Learn how Optimizely One features and CMS benefits can streamline your digital experience
-
March 2025
Optimizely Named A Leader in 2025 Forrester Wave for CMS
Optimizely has been named a Leader in the Forrester Wave™: CMS, Q1 2025. As a Platinum Partner, Mando Group highlights why this matters.